The reserving cycle and how to avoid it
The Casualty Actuarial Society publishes reserving paper using probabilistic programming
The biggest potential blocker to any (re)insurance M&A transaction is reserve uncertainty, particularly when involving recent and/or growing long tail classes. Even the best independent actuaries are forced to rely on generic reserving parameters simply because there may not be sufficient development data from a recently expanded long tail class to form opinions. And because multiple years of business will have been bound before reliable claims development data can be generated, any erroneous judgement made in reserving will be magnified many times over when it comes to a reserve “true-up”, a key feature of the reserving cycle.
So how best to deal with this “READY! FIRE! AIM!” approach to underwriting and reserving new and growing long tail classes when there simply is not sufficient reliable claims development data available?
Well, a recently published paper, co-authored by one of the founders of ICMR for the Casualty Actuarial Society, suggests a new approach based on age-old mathematical principles but conducted in modern probabilistic programming.
Rather than waiting for actual claims development data to materialise (or using generic modelling parameters in the meantime) this Bayesian approach does not wait for data you don’t have yet, but focuses on the data that you do have now: price monitoring data direct from the underwriting teams. Intelligently using the data you do have to help populate and parametrise a new kind of reserving model will give greater confidence in reserves and reserve uncertainty for classes where you still don’t have much claims information. It may also help improve underwriters’ behaviours when it comes to rate change monitoring through providing validated feedback.
This approach uses a hierarchical compartmental reserving model, which captures an insurer’s expertise upfront through a parametric framework for describing aggregate insurance claims processes using differential equations. It then uses emerging claims development data to assess the credibility of those original input assumptions.
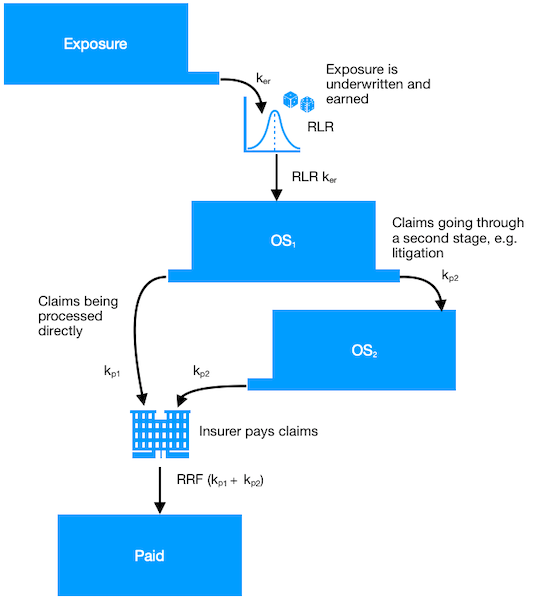
Illustration of a two stage compartmental model, which describes the flow of information or monetary amounts between exposure, claims outstanding, and claims payment states for a group of policies.
But in terms of why this might be of interest today, it could be argued that this lack of a reliable, transparent and mechanical feedback loop between price monitoring and reserve variability modelling is itself a key driver of the reserving cycle. If underwriters really were on top of their price monitoring, long tail reserving “true-ups” would not be as volatile as they are, long tail problems would be identified much sooner than they are and reserve variability would not be the deal blocker it currently is.
For more information please contact Markus Gesmann.